Dynamics-Aware Comparison of Learned Reward Functions
Jan 24, 2022·,,,,,·
0 min read
Blake Wulfe
Logan Ellis
Jean Mercat
Rowan McAllister
Adrien Gaidon
Ashwin Balakrishna
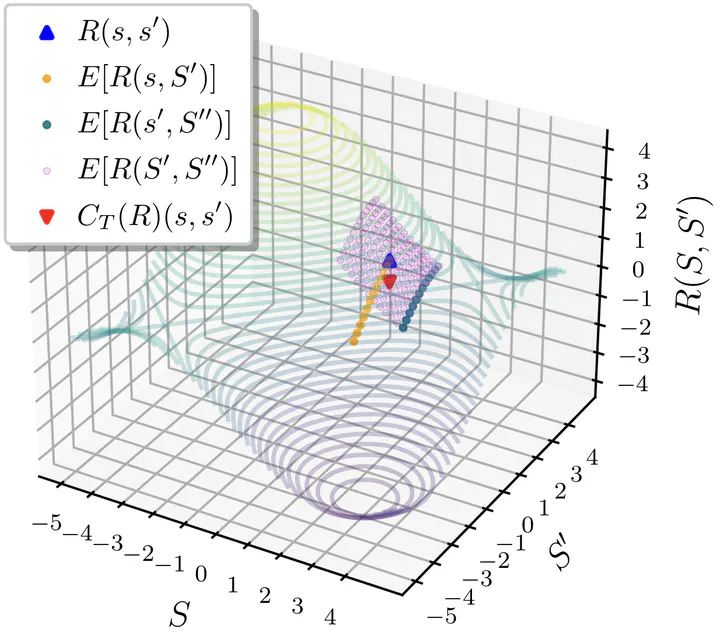
Abstract
Evaluating learned reward functions can be challenging due to their reliance on the policy search algorithm used to optimize them. We propose Dynamics-Aware Reward Distance (DARD), a new pseudometric that leverages a transition model of the environment to transform reward functions for more reliable comparisons. DARD is invariant to reward shaping and only evaluates reward functions on transitions close to their training distribution. Our experiments in simulated physical domains demonstrate that DARD provides accurate reward comparisons without policy optimization and outperforms baseline methods.
Publication
In arXiv